Wasteful Deadly Bias In Fashion Trend Forecast
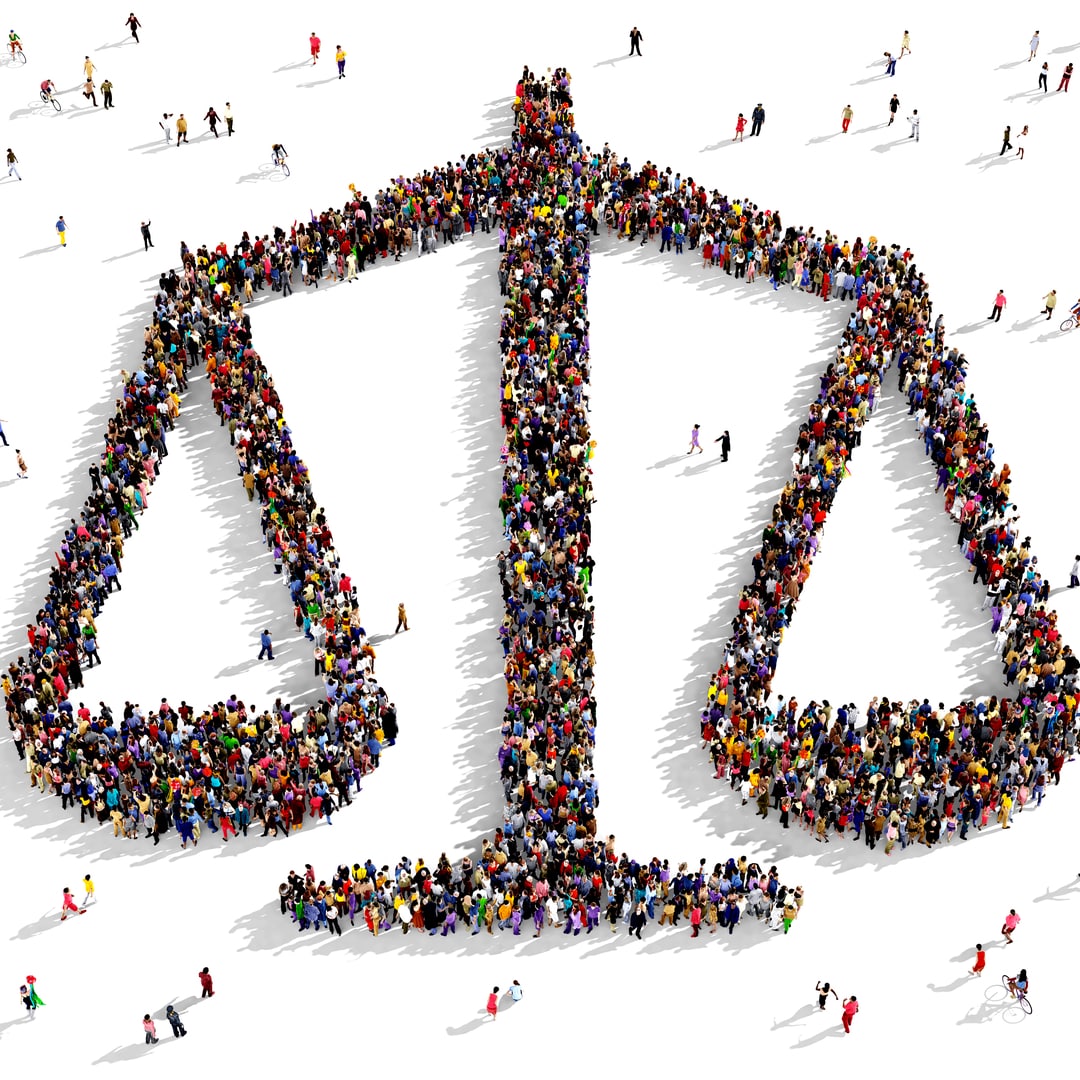
The fashion trend forecast of today has a deadly bias. The amount of fashion over-supply and billions of garments unsold reflects this. This edit makes an attempt to get to the root.
What Are The Decision Biases?
Humans are error-prone and biased. This is one kind of bias, which we normally talk about. We are witnessing data explosion now across all spheres. This creates a lot of data-based platforms which are providing information. These platforms are powered by algorithms. Algorithms are not bias-free.
We make decisions using our experience. Experience essentially is data interpreted through our lens. We combine that with our personal preferences. This creates bias. Training data provides experience to algorithms. Algorithms by themselves are not good. Quoting the Vox article on algorithmic bias,
It’s tough to figure out exactly how systems might be susceptible to algorithmic bias, especially since this technology often operates in a corporate black box. We frequently don’t know how a particular artificial intelligence or algorithm was designed, what data helped build it, or how it works.
Vox article on “A computer can make a decision faster. That doesn’t make it fair.”
The Deadly Fashion Trend Forecast Bias
We start with looking at what does forecast error mean.
Quoting from wiki,
a forecast error is the difference between the actual or real and the predicted
wiki
Fashion forecast error is over 50% or more. As a result, it reflects in the full price sell-through across the industry leaving a small exception. Such a gap is of serious concern considering poor cash flow, declining margins, growing inventory pile in the industry.
We look at this gap through the lens of bias.
Human Bias In Fashion Trend Forecast
The traditional methods of travel, browsing, expert reports are by design with human bias. We see what we want to see. In the book “Liminal Thinking”, Dave Gray talks about how small is our visual perception attention. Our attention is minuscule of what is happening around us. Hence, we have a limit of perception in knowing what is happening. Any cumulation of this a just a small multiplier. However, this is an input for inspiration and not for validation. We can continue to explore but stop before exploiting them with financial resources. Moreover, what we see is what is available which is error-prone and noisy.
This takes us to use data to start informing our decisions. The development of ML/AI (Machine Learning / Artificial Intelligence) systems help us to make sense of data at scale. We will now explore the algorithmic bias.
The Algorithmic Bias In Fashion Trend Forecast
The ML/AI system bias starts with the training data. We see the data based systems in the market for fashion trend forecast, use the data of what is available. “What is available” data, irrespective of scale, has supply bias which is 50%+ incorrect or biased. Any amount of data does not solve this problem. This is fundamental. It is like the foundation of a building. You can’t compensate a poor foundation with any number of storeys. The building will collapse. This is what we are seeing.
For those interested in knowing different biases in any ML/AI project, here is a good article providing 5 biases. Here is another article with inputs on bias prevention.
We will look at the types of data used in fashion trend forecast and their biases.
Types Of Data In Fashion Trend Forecast
We see two primary types of data apart from human inspirations and the brand’s own retail data. The first one is brand, retail data collected from websites. The second one is the data from social media.
Digital Retail Data Bias In Fashion Trend Forecast
The supply of products in digital channels comes with a 50%+ forecast error. This is the starting point of the biggest bias in retail data. Any amount of wrangling of this data won’t get us anywhere. What you get to see are varying information of supply proportions, supply trends, supply prices, supply attributes like color, etc. Stockout signal on its own is not sufficient. There are huge gaps in the quantity forecast by brands in buying products with over and under stock situations.
Social Data Bias In Fashion Trend Forecast
Let us start from the bottom up. How does social influence the retail business? Social gets a lot of attention. The question is how relevant it is in terms of weightage and accuracy to real trends. The impact of social in the purchase should first reflect in their traffic from that channel.
A quick look at the amount of traffic an online and an omnichannel retailer gets through this medium will give us a magnitude of this channel. We looked at ASOS, UK, and Macy’s, US as an example to find out their traffic component coming from social. This is a combination of organic and inorganic actions.
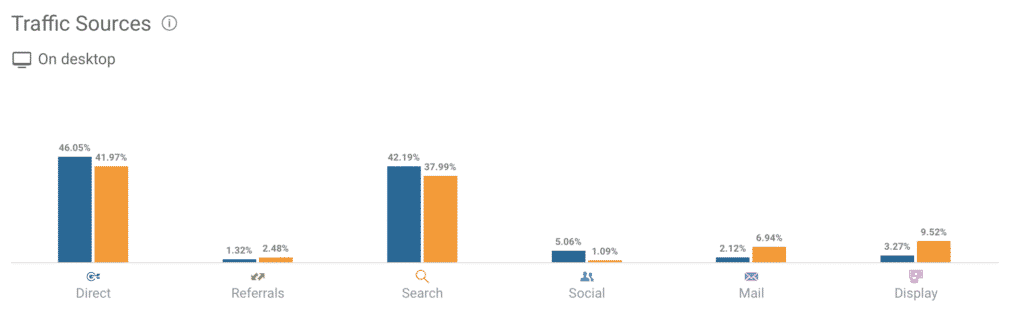
Essentially we are looking at 1-5% traffic across an omnichannel retailer to a digital native retailer. This gives a foundation for weightage.
From an accuracy standpoint, social popularity does not necessarily correlate to commercial success. In our research with clients, we observed an under 40% correlation between social popularity and the commercial performance of products.
How To Mitigate Deadly Biases From Fashion Trend Forecast?
We at Stylumia observed this fundamental cause of the huge supply-demand gap in the fashion industry. We need a consumer demand lens to trend forecast. Today’s lens supplies. This is a non-trivial problem.
This led us to invent Demand Science®. We built an award-winning, proprietary demand sensing engine that removes the noise from the data. This is our fundamental principle across our platform of solutions. This includes market intelligence, buy/plan optimization, and distribution optimization.
We have a unique approach to use the image itself in our prediction models to remove feature bias.
Please check our earlier articles here and here, for a deep dive.
In Conclusion,
- Do not go by the hype of data/ML/AI in choosing your trend forecasting solutions
- Look for the bias in the data and the algorithm
- Use social signals only after sufficient validation
- Look out for bias in how you are reading your own omnichannel data
- Textual attributes are limiting in fashion. They have a role to play. Time to re-think beyond attributes
We are always your fans batting for you to make informed decisions with ever-improving relevance to consumers with accuracy.