Proactive Fashion AI | Demise Of Traditional Fashion Business Model
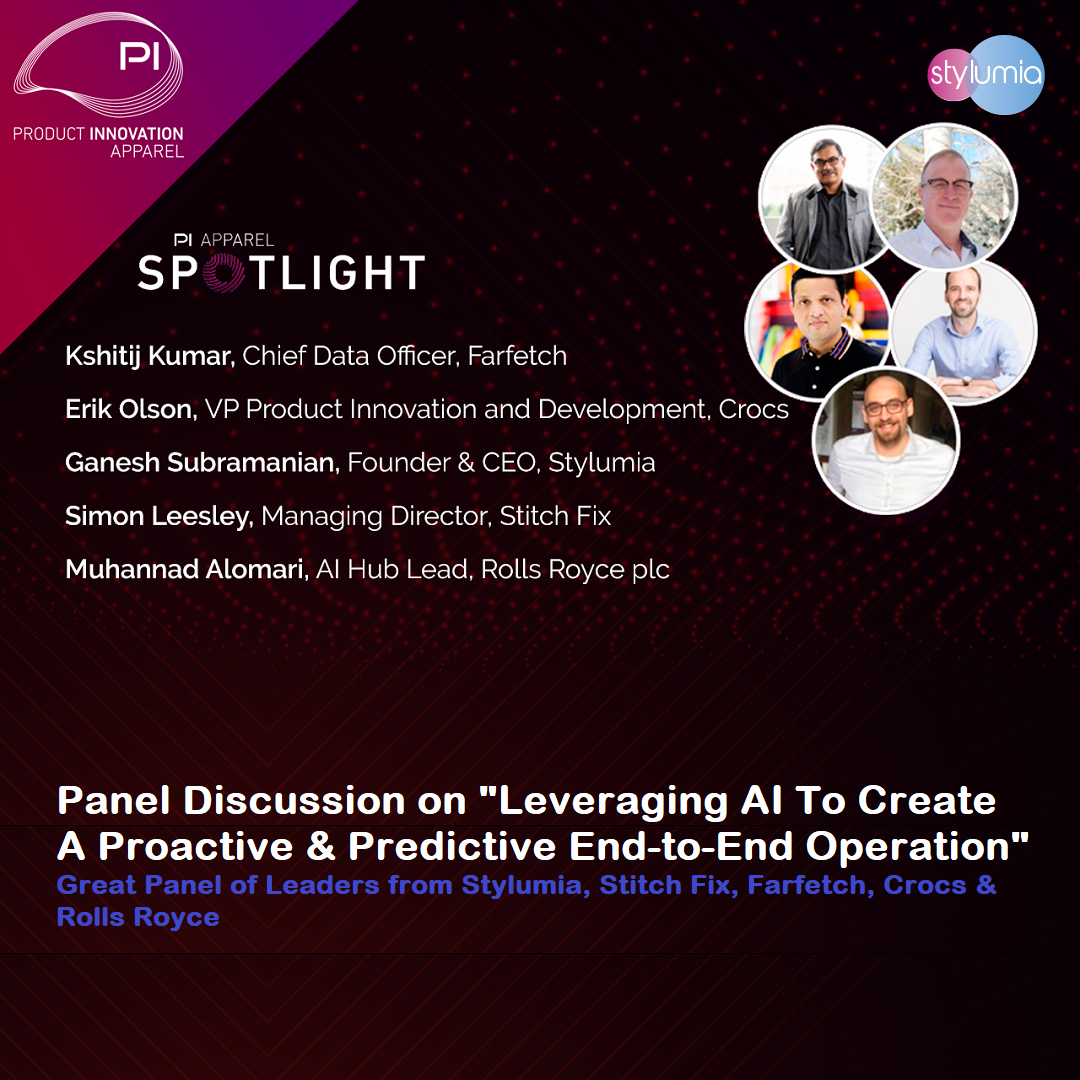
Leaders from Stitch Fix, Farfetch, Crocs, Rolls Royce and Stylumia came together recently to share their views on Proactive Fashion AI for consumers. They also see the demise of the traditional fashion business model. The detailed recording of the panel discussion and the transcript are below.
Full Transcript below:
Setting The Stage For Proactive Fashion AI
David Wilcox:
Hi, everyone, and welcome back. Time for the final session of a jam-packed day. It’s been, yeah, really informative so far and it’s going to get even more so because I’m joined by KK, chief data officer from FarFetch, Erik Olson, VP of product innovation at Crocs, Ganesh Subramanian, who is the founder and CEO of Stylumia, Simon Leesley, MD at Stitch Fix, and Mo Alomari from Rolls-Royce, the AI hub lead. Hello everyone, how are you doing?
David Wilcox:
Fantastic. Good, good, good. Well, welcome to Spotlight on AI in fashion. Let’s start with a few quick introductions. Simon, if I could start with you please.
Simon Leesley:
Yes, thank you. Happy to be here. My name is Simon Leesley. I’m the managing director of Stitch Fix in the UK. For those that don’t know, Stitch Fix is the world leader in personal styling and a personalized shopping experience, where we have multiple experiences where a customer can have a stylist’s handpick items from them, leveraging data science and AI in that process, or they can trust Stitch Fix to deliver a personalized shopping experience that is more curated than what exists in e-commerce today. We have shipped $6 billion worth of clothing sight unseen to customers, using personalization algorithms and human stylists.
David Wilcox:
Fantastic, excellent. Ganesh, for those who didn’t just watch your previous session, perhaps a very brief introduction for you as well.
Ganesh Subramanian:
Hi everyone and nice to be here, and a great panel to be part of. I’m Ganesh Subramanian, founder and CEO of Stylumia from Bangalore, India. We are a deep-tech startup. We enable optimizing inventory through the entire value chain in any fashion brand or retailer by using algorithms right from consumer research, right up to distribution. Happy to be here.
David Wilcox:
Fantastic. Thanks, Ganesh. Mo, over to you.
Muhannad Alomari:
Thank you. Hi everyone, I’m Mo Alomari, and I lead the AI team for Rolls-Royce in the London office. I’m really excited to be here, so I’m obviously not from the fashion industry. I work at Rolls-Royce which is a power system company. We deliver everything from aeroplane engines to nuclear reactors across the globe. We have 50,000 employees again across the globe, 20,000 engineers. Yeah, I’m very much excited to learn more about what AI is happening in the fashion space.
David Wilcox:
Yeah, should be really interesting and great to have someone from outside the fashion industry here as well I think. Erik, a brief introduction for yourself, please.
Erik Olson:
Hi, Erik Olson. I’m in Colorado. I represent the brand Crocs, a global footwear brand. I had worked with a team that a global team that provides everything from product development through sourcing of our product, and we make thousands of decisions every day that are either now enabled by AI or can be improved with artificial intelligence in the future.
David Wilcox:
Fantastic. Last, but very much not least, KK, over to you.
Kshitij Kumar:
Hi everybody, thanks for having me. I’m Kshitij Kumar. I go by KK just because it’s a tough name to pronounce, and I’m actually the chief data officer at FarFetch, FarFetch UK. For those who don’t know us, we are one of the biggest luxury fashion online retailers. We are listed on the NYSE, and we went public a couple of years ago. My job is to basically cover everything dealing with data, so I am on the executive board of the company. I am basically doing everything from marketing analytics to operational analytics, to customer service analytics, artificial intelligence, machine learning, data governance, you name it. If we are doing anything in data, that pretty much falls in my team.
David Wilcox:
Fantastic.
Kshitij Kumar:
Looking forward to this panel.
How Important Is To Be Proactive Than Reactive?
David Wilcox:
Yeah. Well, welcome everyone, one and all. Let’s get started. Also actually, just before that, I just want to remind everyone to get involved in the chat, ask questions, leave some comments on any of the comments that people make here. Let’s get interactive with this one. In light of last year’s disruption and even before that, the demise of the traditional fashion model, how important is it to be proactive rather than reactive, and how has and will AI help companies make this shift? Erik, I am going to start with you on that one sir.
Erik Olson:
Well, thank you. Great panel. I’m really excited. Hopefully, we can spark some good ideas here today. I love the cross-industry piece of it too as well. I think that being proactive as opposed to reactive is always a desire of all business leaders, right? We want to be able to do that. When I look at the future of artificial intelligence in the fashion industry and how we can be more reactive, certainly around things like forecasting and understanding what the consumer wants when much like Ganesh just talked about earlier. Great, great job Ganesh. I also think that there are ways that we can explore creativity with that AI and add more to the consumer value proposition in the future.
Erik Olson:
We’re looking at all different angles, and I think some of the things that are obvious that AI helps with around the forecasting and that type of thing are some of the things that are secondary to the things that we’re investigating with our own supply chain.
David Wilcox:
Brilliant, thanks. Simon, the demise of the traditional fashion model, I guess Stitch Fix has been on the rise of the new one I guess.
Simon Leesley:
Yeah, definitely. I mean I agree with a lot of what Erik just said, and we’re focused on similar areas. I mean one of the biggest ways that we’ve implemented AI in the business that’s helped us be more proactive is on the demand forecasting side. Almost all of our demand is forecast by algorithms with very little human tweaking involved in that. Certainly, this year, when you have black swan events like coronavirus, you have to involve humans a little bit more in that process, but that’s one of the areas that we’ve really dialled in our ability to forecast the business through AI. Then I think the other place where it’s really helpful in our business is the days of long-term planning, buying cycles are gone.
Simon Leesley:
We need to have more continuous agile processing or forecasting in the business. We’re trying to shorten the actual supply chain itself on top of getting more accurate with demand. Using things like our re-buy and re-buy algorithms which essentially recommends algorithmically to buyers when they should be placing additional orders of an item, or when they should consider it in different colors or prints, and then also trying to couple 3D design into that process to put things in front of customers before we ever manufacture them and get reads on those, where we can then algorithmically predict the depths and quantities of which we need to purchase them. All those are really things that we’re already in the pipeline, but we’ve really doubled down on this year given the current environment.
David Wilcox:
When you’re predicting what clothes people should wear, Simon, tell me how hard is it not to get too greedy with how many predictions you send people, and can the data work to give them even more predictions than perhaps you thought were necessary for that particular person?
Simon Leesley:
I mean fundamentally, what we’re doing is we’re using personalization to reduce the paradox of choice and the customers face online today. Our mantra is to put fewer things in front of the customer and leverage a combination of data science and humans to pair down. We have tens of thousands of SKUs in our inventory, but when a stylist goes to pick five items for a client, they only see probably the most relevant 200, 250 for that specific client. All that heavy lifting is done by the algorithms so that you’re not transferring the cognitive load from a human to a human in the customer to the stylist.
Simon Leesley:
Even with our direct shopping feature where clients can go on and see a highly curated e-commerce experience, they see far fewer items than you would in traditional e-commerce, but it’s highly curated to them based on their style profile, their purchase history. Every bit of data that they’ve ever told us about themselves is used to curate that really tight experience for them.
David Wilcox:
Thanks. KK, I’d like to come to you next. The original question was about being more proactive than reactive, and I guess that’s something you guys do as well.
Kshitij Kumar:
Absolutely. I mean I think if you went back and read our prospectus when we were about to go public, we talked about three pillars two years ago, and the third pillar was data. The first one was our knowledge fashion, et cetera, the second was technology, the third was data. We didn’t at that time have a CDO. I wasn’t there. We didn’t have one leader. We weren’t actually doing those things at that time, but we knew what we needed to do. We went out, I came on board. I built a team. Very, very luckily for us, most of that work was done in that fundamental leadership team was there, the fundamental data scientists across the company were all reporting to me.
Kshitij Kumar:
We had started working like one team just before COVID happened, and I must say a shiver went up to my spine when it happened. Apart from everything else, I thought, “My goodness. Are we going to be able to come together as a team and actually work remotely when nobody’s going to see each other for who knows how long?” Thank goodness, everything worked very, very well. Being prepared not just from a technology perspective, but from a cultural perspective. Being able to work remotely, we didn’t skip a beat. In fact, what started happening is data became the way that we could actually see what was happening around the world because we shipped to 190 countries. We have hundreds of thousands of schools in the database.
Kshitij Kumar:
When you start seeing people from China beginning to come back again to the site and start to see certain things, your algorithms, the data models have all actually gone out the window when this kind of an event happens, but then the machine learning starts learning again and start seeing what’s happening. When we started seeing people coming back again, the algorithms actually adapted quite well. We were able to start predicting okay, if this is what’s happening, here’s what we should expect next. I think being prepared for these kinds of things, you can never be prepared for everything, but being in the cloud, making sure your AI, your ML, your data, your analytics, your governance is in place so that you can continue to work through absolutely critical. It’s held us in good stead so far.
Where Part Of The Value Chain AI Is A Natural Fit?
David Wilcox:
Fantastic. Let’s move on because I think there’s some really, really interesting stuff as well. The next question is in which parts of the value chain is AI a natural fit, and there must be some natural fits for it. Mo, I think I’ll come to you directly on that to maybe get an outside industry perspective on that.
Muhannad Alomari:
Well, thank you and first of all, it’s interesting to hear from Erik and Simon and KK how data scientists in different industries have been reacting almost in the same way to the rise of COVID-19. To answer your questions, so there are plenty of basic use cases where AI fits naturally, and that is mainly around when you have decisions to be made and there are huge amounts of data on the back of it that would aid in this decision. Humans are obviously really good at reasoning or connecting pieces of information, but what they are not naturally good at is digesting, or reading lots of data. Just a fun fact, as an activity, we looked at the challenge which is how long would it take a person to read all of Wikipedia, which is not a massive dataset compared to the human knowledge out there.
Muhannad Alomari:
It turns out it would take more than 170 years for a person to read Wikipedia and again in comparison, it took my laptop which is not a fancy machine less than three hours to go through every single character in Wikipedia. That’s where AI fits naturally in use cases. In terms of how we have been utilizing AI in Rolls-Royce, again in response for COVID-19, it’s very similar again to Erik and Simon. It was around understanding the demand that we will have on our product. The civil aviation industry which Rolls-Royce is heavily involved in has been disrupted severely, just like everyone else I guess in the world. We had more than $275 billion worth of losses in this sector, and more than 2.7 million flights cancelled just in May alone.
Muhannad Alomari:
That just gives you a size in terms of how much we have been disrupted, and that meant that we had to adapt and react quickly, and the amounts of data that we were fed on a daily basis is absolutely enormous that no one team in Rolls-Royce could handle. This is where our AI played a major role in helping Rolls-Royce to overcome the financial challenges that we have seen over the past few months, and there’s a lot to talk about there, more there if there is interest.
David Wilcox:
Yeah. What about you Ganesh? What about natural fit? Where does it naturally fit in the supply chain or the value chain? Sorry. I mean I guess you’d say design.
Ganesh Subramanian:
Yeah. I think I agree with Mo in terms of where there is plenty of data and there is a high level of complexity, and more it is unstructured, air comes very handily, right? Just to give you a perspective on the size of the data that we handle. Let’s say we handle retailers like FarFetch, hundreds and thousands of them. For our data size is a superset of hundreds and thousands of retailers data points over a period of time, right? When that’s the level of data, now how do you make sense out of them, right? What’s actually trending? Therefore, if I have to just split the value chain into few parts and say research, buy, plan, and sell and that’s what fashion actually does at a very high level, so it comes in handy in consumer research, right?
Ganesh Subramanian:
That’s one of the areas that we adopt and helped designers make informed decisions in terms of what kind of products to make. I think Simon and others, Erik also talked about where do we take AI into, for example, the secondary spaces wherein creation itself, right? We showcased one of our solutions today about imagining what it does is, it can actually create designs. Now, these inspirations are coming from the face generation. Today, you can create a human face by mixing styles of two people, maybe your hairstyle and maybe your skin color and somebody else’s complexion, right? Now, by using that, we are able to create new designs, and one of the key things in fashion is that we have a huge supply-demand gap.
Ganesh Subramanian:
For prediction, demand forecasting is fundamental here, but the way we do very differently is we use the image itself along with attributes to predict. We put pixels. Therefore, the number of parameters getting into the model is an order of magnitude exponential compared to attribute-based prediction models. Right from design research into prediction is where we use AI, and we have an AI native from day zero. The point is that not just you use AI for the sake of it. There are spaces that where you use traditional models, right? Everything may not be AI, but one needs to be choosy and say which helps the task gets done better.
David Wilcox:
Would anyone else like to come in on that which the differences between different models and approaches? KK perhaps.
Kshitij Kumar:
Sure, I can always jump in. I think just from looking at how wide our approach is, we’re not just looking at the design, or just the operations, or just one part of it, right? If you think about what Google does with Google analytics and things like that or Microsoft, pick Bing, pick Facebook, pick whoever. When a consumer is actually doing an online search to say I want to buy a… I won’t name brands, but pick your favorite brand and say I want to buy something like that, or I’m looking for something like that for Black Friday, for Christmas, I’m looking for a gift for my girlfriend or my wife, that search when that happens there kicks off an entire artificial intelligence chain behind the scenes, right?
Kshitij Kumar:
Google first starts, or Facebook first starts doing what they need to do. We companies like us who are sitting behind, watching what’s happening, we look at it from a different perspective and say, “Hey, what this person is looking for, can we actually sell it to them?” Especially in the COVID times when this happened right in the beginning, right? April, May, we were wondering, can you actually ship it to them, even if they search for it from wherever they are? Can we actually get it to them even if it’s available somewhere in the world, right? All of this behind the scenes is running with artificial intelligence. From getting that customer to the product, wherever that product is. In our case, it’s an online website.
Kshitij Kumar:
Then once they are on the website, what should we show them? What do we want to show them that would be something that they would like, that would make them happy, but also something that’s good for the environment, something that needs to be shipped across the world? If I can also ship something which is a few miles away from where this person is, which one do you want to focus on, right? It’s not just about the profit that the company would make, but how is it going to be for the earth, for the world, and other parameters like that. Then to the points that Ganesh was making earlier, what style? What do I actually show them? Is this something that would suit them, make them happy?
Kshitij Kumar:
Are they going to be able to use it, or is it maybe something that won’t even fit them, for instance, based on past behavior, et cetera? All the way down to the operations. Once the person has bought it, looking at fraud for instance. You put in a credit card, how do you know that this person is real, legitimate, or not and are they the owners of that credit card or not? Going on to when you ship it, how do you tell them you’re going to get this on Wednesday between 3 p.m. and 5 p.m.? Before they’ve even made the order, and you could be on the other side of the earth. Remember, you got to now predict customs when this is going to be shipped, not just aeroplanes picking up the stuff and delivering it, but also the customs guys in multiple countries possibly.
Kshitij Kumar:
AI can be applied to many, many, many things, but the ones where we are seeing the easiest start of course is with things like customer service when somebody picks up the phone and calls or goes online and starts trying to talk to them. Chatbots, but not just chatbots. These are multimodal chatbots now. Have they already done a search online? What did they look for then? What are they asking now? How can I look at it beyond just the one question that they’re asking? What’s the context around it? I think overall, AI can be applied wherever you’ve got complex datasets, data to train from the past, and where you’re trying to do something that is predictable. Even if it’s not predictable for us humans, we may not actually see that it’s predictable, but the machines probably can.
David Wilcox:
Fantastic stuff. I mean I think that’d be really, really interesting to know some of your greatest successes with AI because as you rightly said KK, covers so many areas and it can cover the entire value chain really. Erik, maybe I could start with you on that, and what have been your greatest successes in using AI?
Erik Olson:
Certainly, just that KK said, I think it’s spot-on as the most mature pieces are around marketing and making sure you get your return on investments when you’re marketing and being more targeted. The security piece is another piece that we have longstanding success with, and then the customer service is really an interesting piece because it’s all about being more friendly to the consumer at the end of the day and using that in conjunction with our staff and our people to be more proactive in terms of when customer complaints are going to come and how we can attach those, and then go from there.
Erik Olson:
I think that’s been three areas of success, and I think that to the previous question in terms of those are the most mature areas and that’s where we started as well, I think every company is different though. You have to look to where you have a data set, and you have perhaps a team that’s more inclined to want to use these tools to increase and compound their value to the company.
David Wilcox:
Brilliant. I saw a lot of head’s nodding there. Simon, what would be some of your greatest examples of using AI?
Simon Leesley:
Yeah, I’ll highlight a couple of them. The biggest one is probably in our styling recommendation algorithms. We have an engaging game that we allow clients to play, or ask clients to play called style shuffle. It’s just a game where we show them in our mobile app just hundreds of images of clothes one at a time, and they rate yes or no on them. It’s as simple as that, and that provides just an insane amount of data to train machine learning on. To give you a sense of the scale, we have over three billion ratings from our client base in that game. It allows us to do a lot of things across the business.
Simon Leesley:
First, it powers our latent style algorithms, which is the primary recommendation algorithm that when a stylist goes to style a client, it says out of the 30,000, 40,000 styles that we have, these are the 250 most relevant for this specific client that you’re styling. The second place is it allows us to do interesting things in the supply chain. We can put products that we’re thinking about designing or manufacturing into this style shovel game and get clients to rate it. It actually tells us at that point okay, this is a winning style for a broad base of the customer segment of your customer base, or it might be for this super niche group.
Simon Leesley:
What we can do from there is then essentially recommend how deep we should buy that style and then who to target it to when we actually bring in the product. That’s been hugely helpful for just reducing waste and clearance and markdowns in the overall supply chain, which is critical for fashion. That’s one big one. The other place we’ve had a lot of success certainly on the marketing front, and how we present things to customers. Every interaction the customer has is it’s personalized through AI. After a client checks out as an example where they give us feedback on the items, complete their purchase of what they want to keep and what they want to send back, we have the opportunity at that point to present the client with a lot of different things on the page that shows up after it.
Simon Leesley:
If it could refer a friend if they’ve just had an amazing experience. If they’ve had a poor experience, we could refer them directly to their stylist or customer service. Every touchpoint we have is personalized in some way based on just these massive amounts of data that we have about where a customer is in their like journey, what just happened. Those are things that humans just aren’t good at doing, understanding all those interaction points of huge amounts of data. Those are a couple of examples of how we’ve used it very successfully in the business.
David Wilcox:
Thanks, Simon. Mo, what have been some of your greatest success stories with AI at Rolls-Royce?
Muhannad Alomari:
Yeah. Well, thank you and in terms of the greatest success, over the past two years, we have been heavily involved with the digitization of our supply chain. I guess something was commenting on is what Erik mentioned around you need a team that is also willing to go through the digitization or the use of AI journey with you as they… In parallel, you need the subject matter experts to be as keen, or as motivated to use AI within the organization. Now within our supply chain, it’s a massively over complicated setup that we have that delivers billions of pounds worth of components and equipment, so that we can deliver the products that we do in Rolls-Royce and spread across the entire globe.
Muhannad Alomari:
The slightest change within the supply chain requires massive amounts of analyzing of data to understand the impact that it would have on all of our products. What we’ve seen through COVID or the rise of COVID is that we had a huge risk in terms of not being able to fully understand the impact that would happen by say one of our suppliers declaring bankruptcy, or going into administration, or simply not being able to operate because there are some restrictions within the country or the continent where they sit in. Mapping all of this, as well as creating lots of scenario modelling tools and build optimization algorithms on top of this scenario modelling tool has been by far the biggest value driver for us within the AI team in terms of saving or cutting costs for Rolls-Royce.
Muhannad Alomari:
It was mostly directed around optimizing our supply chain and around well simplifying our supply chain as well. That was the main application for us this year.
How AI Can Drive Scale And Reduce Costs?
David Wilcox:
Brilliant. Thanks so much. Ganesh, I want to come to you next as well and talk about how AI can be used to drive scale and reduce cost across operations. Could you shed some light on that?
Ganesh Subramanian:
Yeah. Again, we are a B2B enabler. Our customers are brands and retailers. One of the fundamental areas is let’s say research, if it’s a private brand retailer, or if you’re a retailer with multi-brand, and you’re spending a lot of time and energy in buying. We cut down the research time by 75% before Forex increases the reduction in research time. What that means is increase significantly time to market, right? You’re not actually all the time-wasting time invalidation, but we quickly validate. Therefore, that’s one speed to market is going to be very critical. Second is whatever decisions you are taking, you need to take them right. The area that we help and the metric that we move for our customers is basically full-price sell-through.
Ganesh Subramanian:
How much of your products are you selling through in the system, through the sellout in the system? Whether you do it through retail or through wholesale, how do you do that? That’s something that we shift anywhere between 20% to 30% shift in the full-price sell-through. coming to the intensive operation space which is the quantitative side of the business, we normally say there’s no bad product if you bought the right quantity, right? Therefore, it’s very fundamental and the question here is people miss the quantitative forecast a lot of fashion in the qualitative side of it, and quantitative side of prediction getting the depth right and your width of options right is critical to getting the overall.
Ganesh Subramanian:
Particularly in sustainability right now, there’s so much wastage, right? We so far eliminated close to 60 million garments and of course, we are growing and they still grow with us. How do we minimize that wastage? That’s what we do in the buy optimization. In distribution, I think very interesting and what we have seen is through COVID, our models are able to adapt very, very quickly, right? For example, let’s say the offline stores have started to pick up slowly while the online was growing faster, and predicting a store in this dynamic environment was challenging. What we could see of course, the model did not work in the first few weeks.
Ganesh Subramanian:
Within the third, four weeks, we could see that our models are adapting to the COVID situation and as the stores open, it was correcting online demand also. In prediction terms, there is a technology called mean absolute percentage error. We were able to get a map of just 4% to 5%, which means that we’re able to get demand accuracies through the COVID period close to 95%. Now because of that, I think our clients start to get confidence, right? With COVID, people actually start getting very conservative. My demand will be very low, they were not placing enough bets. Once the accuracy started improving, they started placing bets, right? They started to believe the data. Now today, sales are increasing.
Ganesh Subramanian:
I think when we say prediction, it’s actually conservative, effectiveness, all of that. What we are saying is prediction can actually completely magnify the business. It can be a revenue growth driver. It builds a lot of optimism in the decision-makers. That’s what we see across the value chain and eventually resulting in sustainability.
How To Prevent AI Creating A Narrow Focus?
David Wilcox:
Fantastic. I see a lot of nodding heads there as well. Guys, we’ve gone through half an hour already. It’s flowing by, but look, we’ve got another half an hour. We’ve got loads of questions coming in. I’m going to get into them, and I guess I’ll try and direct them to the appropriate person, but if anyone wants to come in on it, just either raise your hand or just jump in. Right. AI is known for sending people down a rabbit hole of narrower and narrower focus. How do you prevent this and allow people to see new things and have people open to new options for purchase? I’m going to go with KK first and foremost.
Kshitij Kumar:
Sure. I think that’s a great question. Obviously, it’s coming from somebody who’s a practitioner because this is a real problem. We see it, it does happen, but I think the way that you have to avoid it really is by making sure that your datasets and what’s going into making that decision is not the same old, same old, and that there’s also a feedback loop. What you have to have is if you’re actually sending people down into a narrower, narrower set of things, you’re going to run out of those things. If it’s let’s say, it’s just a ranking algorithm, let’s talk about ranking, right? Your search for jeans on our website, you probably see 10,000 types of jeans.
Kshitij Kumar:
Now if you always keep recommending the same top 10 jeans, then those are going to sell out right away, and you’re never going to be able to satisfy everybody. It’s not a positive experience for anybody. What you have to do is a couple of things. One is obviously, you have to make sure that you’re getting the feedback. If you only have 10 of those jeans and five of them have sold out because of what’s happened in the last few days, then you have to know that you cannot recommend that again.
Kshitij Kumar:
You have to first change it based on what’s actually happening on the website, but the more important one in our case, the website, the more important one though is to bring in other datasets to make sure that the decisions… The machine learning algorithms will only go towards the data that they’re seeing because they’re training themselves. They’re learning from what you’re giving it. The way we avoid that is you have to make sure that you actually get a broader selection of data, not just for instance even data from your own website, what can you get externally and how do you change that? How do you change some of the parameters in the model? You have to watch the model over time as well to see if it’s deteriorating or not.
Kshitij Kumar:
There are lots of technologies and techniques out there for making sure that your model is not misperforming, but I’m happy to hear what some of the others are doing.
David Wilcox:
Yeah. Mo, would you like to come in on this? I think it’d be really interesting to hear your thoughts.
Muhannad Alomari:
Yeah. Well, thank you and great, great points KK and also yeah, great question. For us, it’s a mixture of thoughts on this. The first one is obvious, when you have safety-critical systems, like some of the ones that we are building, you actually want your data scientist to be really deep into the understanding of the intricacies, or the details of what’s happening in your data because well a lot of the results that will come from the AI system that we are building will impact the decisions being made. For example, one of our more mature programs is called EHM or engine health monitoring. It’s been going on for 30 years. It’s conjugal of data analytics in Rolls-Royce, and we have hundreds of data scientists working on this for 30 years.
Muhannad Alomari:
This is one example where AI is delivering huge values in terms of distinguishing or creating a difference between the safety of the products from Rolls-Royce against our competitors. It’s seen as an advantage that you’re actually becoming really knowledgeable within that one specific area. Now obviously, the counterargument as KK puts as well is you’re missing out on a lot of what other data says, or what other research is going on in other areas. If you become too involved in one sector, or in one problem, or using one algorithm, then you’re missing out on a lot of exciting and also beneficial research happening within other areas.
Muhannad Alomari:
Just as an example, we for example now run in terms of how do we mitigate this. We run bi-weekly sessions in Rolls-Royce, inviting all different data science groups within the company to talk about AI that is happening from outside of the engineering domain. For example, the bioinformatics or the pharmaceutical industry have been doing some really exciting research in terms of mining knowledge from millions of papers and patents and books to help design speed up the design of medicines, which obviously is very relevant now for us in the time of COVID-19. The thought that we had is how can we perhaps repurpose, or borrow some of that thinking in terms of can we use this system to help design better engineering systems and for Rolls-Royce.
Muhannad Alomari:
Yeah, it’s again double-sided. You want your teams to be really good at what they do because it really matters that they’re doing it, but also you don’t want them to miss out on all the great stuff that is happening out there.
David Wilcox:
Yeah. Would anyone else like to come in on that how to avoid this rabbit hole scenario of narrow and narrow focus? I don’t know, Erik, Ganesh, or Simon, have you?
Erik Olson:
I think what Mo was describing there is getting different people to look at it because it is a great question. It is both sides the thing boiled down precisely is that you have to edit to be precise, but I think we think about this a lot in terms of Crocs. It’s just the fact that I don’t think any machine learning would have ever predicted a clog, a plastic injected clog being as big as it was. We’re looking at that. It is about bringing it back to the people and letting people look into it, and uncovering the biases that might be there. As much as machine learning does for us, I think it’s also important to include your people in these decisions.
Simon Leesley:
Yep. I’d agree with a lot of the points that have been made, and one of the benefits we have in our business is that we have an actual human in the middle of the process with the stylist being the last mile of selection for the customer. In some instances, the stylist is overwriting what the algorithm is recommending because of some qualitative feedback that the stylist or the client might have left. They might have said, “I have a wedding coming up and I need you to send me a couple of dresses.” Now an algorithm isn’t going to be great at processing that, and that’s where a human can override the recommendations.
Simon Leesley:
We’re always like we have that human piece that is seeking to constantly train and retrain algorithms based on some of these other factors that may be at play. That’s one of the ways that we overcome it, is always having humans involved in the selection process as well.
David Wilcox:
Okay.
Ganesh Subramanian:
It’s a wonderful question. We see this in action. Let’s imagine a brand that has the data of past data, and let’s say that they have not made a particular tone of red, right? Now when they are making a new decision on a product, you are making a new color which you’re never made, how do you make the decision? Because the model does not have data for which you are now taking a decision, right? Now I agree with KK. I think one way to look at this problem is an explore the exploit problem I would call it in generic terms. Now I think most of the AI algorithms are all fed by data. Therefore, the data bias gets into the outcome. One way to minimize the data bias is to enrich the data and increase the diversity of the data, right?
Ganesh Subramanian:
Now how do you do that? For example, let’s say, if a brand has only limited data, what we do is enrich with all the related markets. It may not be even fashion, right? Now the question is we can bring alternate data. How do you bring alternate data into the model, so that the model is getting richer and richer? Now I think it’s a topic we can keep on discussing, but the question is to bring diversity. I really like Simon’s point of view in terms of how can human bring that value. I think in the context of AI, this is where humans can make a huge difference, right? Now we need to bring diversity into what we are training these models with. I would call all of us to be AI trainers. Now let these be trained by us, right?
Ganesh Subramanian:
Therefore, we are controlling them, and we give that diversity. We give that distinct point of view to explore the outcomes. Yeah, wonderful, wonderful points. I really enjoyed all the points of view here.
David Wilcox:
Yeah, yeah, it’s really interesting stuff. I mean one of the questions is maintaining a human touch important to your business. I mean, of course, it must be, right? If so, how do you complement creativity and emotion with artificial intelligence, instead of undermining it? I don’t know who would like to come in on that because I think it’s a really, really interesting question as well. How do you complement creativity and emotion with AI, instead of undermining it?
Simon Leesley:
I can jump in there, take a lead on that. I mean it’s central to everything that we do and we think about our use of AI as art and science in every function in the company. It’s focused on what do machines do best and what humans do best is the question that we fundamentally ask ourselves. Where there’s typically emotion involved or relationships with clients involved, that’s where humans tend to be better. One of the biggest areas is we don’t want a stylist selecting from 20,000 to 40,000 styles for a client.
Simon Leesley:
We want them selecting from a much more curated set, but they’re really playing that last mile of taking the natural language, which machines still aren’t great at today and curating that final experience for the customer, but it’s also in our buying process, we have algorithms doesn’t make recommendations, but we still have a human oversight of that because there are factors that the buyer might know about that an algorithm might not. That leopard or animal print is going to be a big trend for the season. If that hasn’t been a historical trend, an algorithm isn’t going to know that or predict that necessarily based on the data that we have. It might be in some of the tools that Ganesh has.
Simon Leesley:
That’s where you still need that human piece to provide a little bit more judgment on top of what the algorithms are already recommending.
David Wilcox:
Thanks. Mo, would you like to comment on this?
Muhannad Alomari:
Absolutely, yes. I think you raised a really exciting point there Simon, which is the narrowing down of options from 40,000 to a more focused set. We have been experimenting with this actually within engineering. Engineering is unfortunately sometimes seen as not necessarily within art, but a lot of what we do has an artistic view to it. When you are presented with infinitely viable options, which one do you go to? This is where the most experienced engineers in the organization have this feeling to say, “All right, I know we should head in that direction and that’s where the answer is most likely to be.” We have been experimenting with a concept called generative design, where basically the engineer is designing the problem, rather than the solution.
Muhannad Alomari:
Then an AI algorithm experiments with millions and millions of different designs and coming up with the most optimized one. What you typically see within these designs, if you were just to Google this, you will see a lot of 3D models or shape that come out of this AI that looks very exciting, very natural. They’re not like sharp edges and corners, and they actually perform far better in terms of optimizing for weight or cost or manufacturing time. This is an exciting area for us and yeah, generative design has been used in generating art before. We are now looking at how do we make use of it within the engineering space.
Is Generative Design Used In Fashion?
David Wilcox:
I think that’s fascinating. It’d be interesting to know if people have been using generative design in fashion. I don’t know if KK or Ganesh, or anyone knows about that, Ganesh.
Ganesh Subramanian:
Yeah. In fact, we are just getting into the market. This is I would say an area where creativity is of the highest order, and we also wanted to be very careful in terms of how do we find and use cases for AI in the creator space. The approach we have taken with imagining which you all have seen is how do we help the designers basically get a shortlist of ideas, which they can actually go to market very fast with. It’s not about replacing anything, it’s about augmenting a creator’s ability to come out with designs much faster, and also get a visual perception of the demand, right? We can actually give a concoction of attributes and say please make designs using these attributes, where there are millions of interpretations of those. Instead, we are giving visual cues.
Ganesh Subramanian:
Now imagine his job is to come out with a lot of visual cues, which creative people could use to take it forward and also, it could be used for testing prototype, testing put it out in the market and get feedback and various ways that we could look at exploring these early stages.
David Wilcox:
KK, could you come in on this? I guess there’s a few things here from the human touch to generative design.
Kshitij Kumar:
Yeah, I think our customers, I didn’t mention is we own some brands ourselves, some of the world’s most interesting newer brand…
David Wilcox:
Oh, I think KK might…
Kshitij Kumar:
… as part of our company and what our customers are looking for when they’re buying these things is they’re looking for that emotion. They’re looking for that real creativity that comes. When you’re spending a significant amount on purchasing something of really high quality, it’s not just the product. It’s what it makes you feel, and that feeling today is not possible to be generated by AI, at least I haven’t seen it yet. We find that in our higher-end brands, it’s mostly humans who are actually coming up with what that should… points that everybody else made. Selecting some of those things and reducing the amount that the human has to see that yes, absolutely, we can do that with AI, but when it comes to actually do that creative part where that emotion gets involved, so far, we’re not there yet.
Kshitij Kumar:
It’s humans and it’s not just humans. These are humans who’ve really been doing this for a while and really understand the customers, and how this slight change in that product is going to make their customers feel, and that part, AI isn’t there yet, I don’t think.
David Wilcox:
I wonder if we want to get there, do we? I don’t know, Erik or KK, what do you think? Do we want to get there?
Erik Olson:
For me, I think we do want to get there. I think unleashing and creativity is the most exciting thing. To draw a clear example is just I’m an old guy, so we’ve been working in 3D printing for years and years. Early on in the engineering realm, we use 3D prints to recreate the things that we are eventually going to manufacture, so we can get a prototype and those type of things. Just like Mo said, the generative design of what you can create more organic shapes and those types of things, it’s a big unleash. I get really excited when I think about the engineering problems of solving which there are great examples from brackets and aeroplanes, and all these types of things, but when you look at what it can do in the fashion industry, it’s unlimited.
Erik Olson:
You have to walk that fine line of curating what you want to look at, but then being able to give the designers a spark because sometimes, it’s… I remember the first time we 3D printed a shoe and the organic shapes we got, and those types of things. It really created or made me think of the different things that we could do with this. We’ve used that generated design in the test phase which I think Mo brought up or maybe it’s Ganesh, but we’re able to 3D print a shoe that actually is 100% the same weight, feel, cushion as the final product. Now how we take that into to let our designers explore more creative solutions, that’s the most exciting thing that I see with AI.
David Wilcox:
For sure. Brilliant. We’ve got about…
Kshitij Kumar:
I’ll actually take a contrary-
David Wilcox:
Go on. Oh, go on. Yes, please.
Kshitij Kumar:
…, a contrary and view to it. It’s no fun when we all agree, right? I’d say actually I don’t want us to get there, and I’m actually afraid of getting there at some point because maybe I’ve watched too many science fiction movies, but I want humans to have that edge in what we create, and I want us to be able to actually add what is art otherwise if computers can generate the same thing. Then will it actually create that feeling inside us and our customers? Where I do agree with what Erik is saying is when, for instance, if we can get these chatbots to correct. I lived in Silicon Valley for 20 years, and then I moved to Berlin for a couple, and I just moved to London a few months ago.
Kshitij Kumar:
I moved into this place and obviously, the people that I talk to on the phone to get my internet fixed don’t know that I’m an engineer with 30 years of experience, been building telco stuff for a long time and I know exactly what I’m doing when I’m testing these things. I’m on the chatbot, or even on the phone talking to customer service, and the customer service person just follows a path, tries to follow, go through this thing and they will escalate it after that. What I would love is for a chatbot that really was multimodal, really understood as soon as I typed a few things in, they know okay, this is not a person who is just playing around with this thing, he knows what he’s doing and he’s obviously done X, Y, and Z.
Kshitij Kumar:
Understand it and then immediately get me to that next level, and give me the real support that I need. That level of intelligence isn’t there yet, but that would really help if we had for customer service, for instance, really solve my problem. I don’t have time, don’t waste my life, get me to the answer right away.
Your Dream Use Of AI
David Wilcox:
Yeah, absolutely. Well, I think that’s a really nice thing to ask the rest of the panelists as well, what would be your dream use of AI for the future that isn’t happening right now or isn’t plausible in the next six months? Where would you really, really want to get it too? I’m choosing who to go for first here, who’s going to get the short straw. What about you Ganesh, I will ask you to explain maybe what your dream use of AI would be.
Ganesh Subramanian:
Yeah, let me get out of my business, et cetera. Let me just talk generally. I was just going through an interesting reading on GDP is not a measure of well-being. We’ve all been measuring something which is called capitalistic point of view. Real well-being is about when sustainable development of the world, right? How do we use all of this cutting-edge technology to really improve the real well-being and satisfaction of humans which is happiness, right? I would like to see it. For me, the dream is that all the technology is going to non-trivial problems which are fundamental to the core of co-existence, eliminating poverty, getting into well-being and making a planet that is sustainable. I would put that on top ready.
David Wilcox:
Brilliant. Mo, what about you, what would be your dream scenario? I mean it’s pretty hard to be saving the planet that Ganesh puts down, but how might your dream use of AI go?
Muhannad Alomari:
I have a more specific one. Just some background, so for anyone who has a PhD in mathematics, there’s a family tree where basically your supervisor is considered as your parent and then his supervisor or her supervisor is considered the grandparent. If I go back six generations away, Ludwig Boltzmann, who is one of the founders of fluid mechanics is basically my great, great, great, great, great grandparent in mathematics. He started a field of fluid mechanics that remains unsolved till today. It’s actually one of the longest or it’s probably the longest standing mathematical problem that remains unsolved in the world of engineering and mathematics.
Muhannad Alomari:
I see AI being an enabler that would someday hopefully help us uncover, or have a better understanding of how the nature of physics and life happens in this world. I see AIs as a way to help us understand that better.
David Wilcox:
Thanks, Mo. That’s really good. Simon, I’m going to come to you next.
Simon Leesley:
Yeah, I think one of the most interesting for me is in the wearable tech space and the connection to medical outcomes, and being able to just monitor everything that’s happening with somebody, and using AI to essentially flag issues before you might go to an emergency room, or go to a doctor yourself. I think it’s just that ongoing evolution of wearable tech and the interface with algorithms and AI to how it can make us all healthier and safer and better predict issues before they come up. That’s where I would hope it goes.
David Wilcox:
Fantastic. Erik last, but not least, what do you reckon?
Erik Olson:
Well, that’s hard to follow there. I mean we have really good ideas, and I’ve aligned with all those I think. For me, if I wanted to solve all those problems, it’s all about engaging your employees. If we use these as tools that age-old thing is it’s going to remove jobs, I think it’s going to enhance jobs. If we can make employees more engaged and be a little bit smarter and a little bit more creative, then the problems like health, fluid dynamics, and sustainability I think will be easier solved with more engaged employees.
David Wilcox:
It’s fantastic. I guess well, the greatest challenge with AI is humans than in that case. On that KK, what would be your maybe final thoughts with regards to the use of AI now and in the future?
Kshitij Kumar:
I think we are at a very, very good place right now. We are lucky actually to be part of this revolution because what has happened so far is just the fundamentals. What’s going to happen, Erik, your point was actually right, we are going to get there at some point regardless of whether we should or shouldn’t. I think we are at such a nice place today that if we all do the right things today, we will probably solve the questions, the comments that Ganesh made and Simon made, and all of us made. We will get there for sure. The important thing for us is A, how can we help each other get there if you think of it as competition in some ways maybe, but as a wise man said, if I have seen far, it’s because I stood on the shoulders of giants.
Kshitij Kumar:
We are in that lucky situation that we have the giants here now, and we can all work together to really take AI to where it can get to for the good of all of us, our businesses as well as humankind. I think there’s a lot going to happen. We’re lucky to be in this part, let’s take advantage of it.
David Wilcox:
Thanks, KK, brilliant. Mo, over to you, some final words?
Muhannad Alomari:
Well, first of all, thank you for having me. It’s been really exciting to hear about what others are doing in the AI space within other industries. I had lots of thoughts, lots of good questions where there is from this session that I look forward to discussing with my colleagues in Rolls-Royce internally. Yeah, and as KK said, the future looks really exciting, so I’ll be looking forward to hearing more about what’s happening within the fashion industry in the AI space.
David Wilcox:
For sure, sure well. Yeah, we’d like to thank you as well for coming and sharing your experiences and thoughts from outside the industry. It’s always really useful to get that. Simon, what would be your last comments on this topic?
Simon Leesley:
Yeah. Again, thank you for having me on the panel and for everybody on the panel. It’s a really interesting discussion, and a lot of good takeaways I think for applications in our business, but I agree with KK. I think we’re at a very interesting point in the evolution of AI and what’s to come in the future, so many exciting applications in industries where we’re just scratching the surface of it. I’m just very excited personally for the future of what it’s going to bring and how much better it will make our lives in humankind.
David Wilcox:
For sure. Erik, if you would as well.
Erik Olson:
Yeah. I love KK’s point. I think if we all work together, this thing becomes more powerful and I think it’s not always all about the competition because there are things that we can do to make a more sustainable ecosystem within the industry, and then learning across industries that are so valuable, and that’s what makes events like this so great. Thank you for inviting me.
David Wilcox:
Yeah. Well, we really appreciate your participation as well. Ganesh, if you would close this out, what are your final thoughts?
Ganesh Subramanian:
I think it was fantastic learning from all the panelists here. I have more points noted down than how much I’ve shared. Thanks to each one of you, and the point here is I think this is the time for collaboration. I completely agree with everybody. This is a time for collaboration, participation, and I just want to leave somebody… I’ve been in the industry for a while, and one of the CEOs told me that I am a brand, my competition, both of us have a same-store, a store in the same high street. He makes an LO, I make an LO. It doesn’t sell well and we don’t share our data. Now, the point is I think this is the time for sharing, this is the time for benefiting everybody with collective wisdom, and I really concur with all the points of view towards the end of this session, and thank you for the opportunity.